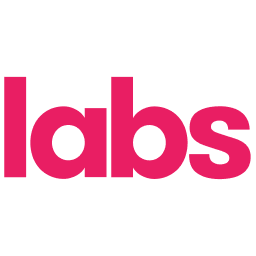
Jamba 1.6 Mini
AI21 Labs
AI21’s Jamba Mini 1.6 redefines what an open-weight, mid-sized model can do—especially in long-context reasoning. With a hybrid architecture combining Mamba (state space models) and Transformer attention, Jamba Mini activates 12B parameters (from a 52B MoE) and supports a massive 256K-token context window. This positions it far ahead of peers like Mistral 7B and LLaMA 3 8B in retrieval-augmented generation (RAG) and grounded QA. Enterprise deployments report standout gains: Fnac saw a 26% quality improvement over Jamba 1.5 Large while slashing latency by ~40%; Educa Edtech achieved >90% retrieval accuracy; and one digital bank matched GPT-4o’s precision using Jamba Mini—with full on-prem privacy. What sets Jamba Mini apart isn’t just performance—it’s efficient utility. The model handles structured JSON output, multilingual tasks across nine languages, and tool use with native support for Hugging Face’s Tool Use API. It delivers up to 2.5× faster inference than dense models of similar size, enabled by its MoE-Mamba backbone. While official benchmark scores for the Mini variant are still emerging, its design choices and early real-world metrics make it arguably the most capable and deployment-ready open model for high-token, high-recall use cases in enterprise AI.
Model Specifications
Technical details and capabilities of Jamba 1.6 Mini
Core Specifications
52.0B Parameters
Model size and complexity
256.0K / 256.0K
Input / Output tokens
March 12, 2025
Last 30 DaysRelease date
Performance Insights
Check out how Jamba 1.6 Mini handles various AI tasks through comprehensive benchmark results.
Detailed Benchmarks
Dive deeper into Jamba 1.6 Mini's performance across specific task categories. Expand each section to see detailed metrics and comparisons.
Non categorized
Arena Hard
CRAG
FinanceBench
HELMET LongQA
LongBench
Providers Pricing Coming Soon
We're working on gathering comprehensive pricing data from all major providers for Jamba 1.6 Mini. Compare costs across platforms to find the best pricing for your use case.
Share your feedback
Hi, I'm Charlie Palars, the founder of Deepranking.ai. I'm always looking for ways to improve the site and make it more useful for you. You can write me through this form or directly through X at @palarsio.
Your feedback helps us improve our service