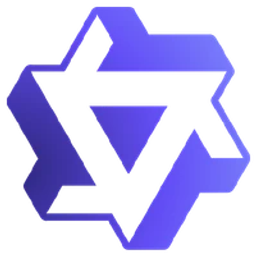
Qwen2.5 7B Instruct
Qwen
Qwen2.5-7B-Instruct is a 7 billion parameter language model finely tuned to master instruction following. It demonstrates exceptional proficiency in creating extended content (over 8,000 tokens), interpreting structured information, and producing structured output formats such as JSON. This model offers improved performance in mathematical reasoning, coding tasks, and multilingual applications, supporting over 29 languages, including Chinese, English, French, and Spanish.
Model Specifications
Technical details and capabilities of Qwen2.5 7B Instruct
Core Specifications
7.6B Parameters
Model size and complexity
18000.0B Training Tokens
Amount of data used in training
131.1K / 8.2K
Input / Output tokens
September 18, 2024
Release date
Capabilities & License
Performance Insights
Check out how Qwen2.5 7B Instruct handles various AI tasks through comprehensive benchmark results.
Model Comparison
See how Qwen2.5 7B Instruct stacks up against other leading models across key performance metrics.
Detailed Benchmarks
Dive deeper into Qwen2.5 7B Instruct's performance across specific task categories. Expand each section to see detailed metrics and comparisons.
Math
GSM8K
Coding
HumanEval
MBPP
LiveCodeBench
Knowledge
MATH
GPQA
Non categorized
MMLU-Pro
MMLU-Redux
MultiPL-E
IFEval
AlignBench
LiveBench
Providers Pricing Coming Soon
We're working on gathering comprehensive pricing data from all major providers for Qwen2.5 7B Instruct. Compare costs across platforms to find the best pricing for your use case.
Share your feedback
Hi, I'm Charlie Palars, the founder of Deepranking.ai. I'm always looking for ways to improve the site and make it more useful for you. You can write me through this form or directly through X at @palarsio.
Your feedback helps us improve our service